When engaging in extended interactions with ChatGPT and other similar artificially intelligent chatbots, it becomes evident rather quickly that falsehoods can be espoused.
Termed as hallucination, confabulation, or a mere fabrication, this issue has now permeated the realm of every business, organization, and even high school student relying on generative AI systems to produce documents and accomplish their tasks.
The ramifications of this problem extend to scenarios with potentially far-reaching consequences, ranging from the field of psychotherapy to the painstaking process of researching and writing legal briefs.
With such critical applications at stake, the prevalence of this issue has become a cause for concern.
According to Daniela Amodei, co-founder and president of Anthropic and the brains behind the chatbot Claude 2, there appears to be no AI model available today that remains immune to the tangles of hallucination.
These inaccuracies and fabrications can undermine the integrity and reliability of AI-generated content, posing significant challenges in ensuring the veracity of the information provided.
As businesses and organizations increasingly rely on AI technologies for decision-making processes, the presence of hallucination within these systems becomes an obstacle that must be overcome.
The consequences of this issue extend far beyond the existence of inaccuracies in AI-generated content. In sectors such as psychotherapy, where patients rely on AI chatbots for support and guidance, exposing individuals to erroneous or fabricated information can have detrimental effects on their mental well-being.
Similarly, in the legal field, the production of accurate and dependable legal briefs is of utmost importance, as it directly impacts the outcomes of cases and justice itself.
If chatbots are prone to generating falsehoods, the potential for misrepresentations and miscarriages of justice arises, highlighting the urgent need to address and rectify this hallucination problem.
Efforts are being made by researchers, developers, and AI experts to tackle this issue and minimize the prevalence of hallucination within AI models.
Numerous methods are being explored, such as fine-tuning models on specific tasks, implementing robust fact-checking mechanisms, and incorporating adversarial training techniques. However, it is evident that a definitive solution remains elusive.
In conclusion, the pervasiveness of falsehoods and hallucination within AI chatbots has become a significant concern within various domains.
Whether it is in the context of businesses relying on accurate and reliable information to make informed decisions or individuals seeking support and guidance from AI-driven psychotherapy chatbots, the presence of fabricated content poses serious challenges.
As the impact of AI technologies continues to expand, urgent and concerted efforts are necessary to minimize the occurrence of hallucination and ensure that AI systems can be trusted sources of information and assistance.
According to Amodei, the primary purpose of these models is to anticipate the subsequent word in a given context.
However, it is important to acknowledge that there will inevitably be instances where the model’s predictions are inaccurate. The accuracy of these predictions is subject to a certain rate of error, which is inherent in the nature of the models themselves.
Despite their impressive capabilities, it is crucial to recognize that these models are not infallible and may occasionally produce incorrect or misleading results.
This inherent limitation emphasizes the need for cautious interpretation and critical evaluation of the outputs generated by such models.
While they undoubtedly offer valuable insights and assist in various tasks, it is essential to exercise discretion and not blindly rely on their predictions without thorough scrutiny.
Anthropic, ChatGPT-maker OpenAI, along with other prominent AI developers specializing in large language models, is currently engaged in concerted efforts to enhance the veracity of their systems.
Nevertheless, the timeframe required for achieving this objective, as well as the ultimate viability of these models in dispensing trustworthy medical advice or similar tasks, remains uncertain.
According to Emily Bender, a distinguished linguistics professor and the director of the Computational Linguistics Laboratory at the University of Washington, this issue is not easily remedied.
She believes that the dilemma originates from an inherent disparity between the technological capabilities and the proposed applications, thus posing a formidable challenge.
The reliability of generative AI technology holds immense significance, as it has the potential to contribute trillions of dollars to the global economy.
According to the McKinsey Global Institute, this technology could add anywhere between $2.6 trillion to $4.4 trillion to the world economy. The applications of generative AI extend far beyond chatbots, encompassing a wide range of tools that generate images, videos, music, and computer code, almost all of which involve a language component.
Notably, Google is already promoting an AI product for news-writing, recognizing the criticality of accuracy in this domain. The Associated Press has also joined forces with OpenAI to explore the utilization of generative AI, with OpenAI harnessing a portion of AP’s vast text archive to enhance its own AI systems.
In collaboration with India’s hotel management institutes, Ganesh Bagler, a computer scientist, has devoted years of research to develop AI systems capable of inventing recipes for South Asian cuisines.
One of Bagler’s previous projects, a precursor to ChatGPT, focused on concocting innovative variations of rice-based biryani. Interestingly, the success of these AI-generated recipes hinges on accurately identifying the right ingredients.
A single “hallucinated” ingredient could be the determining factor between a delectable dish and an inedible meal, underscoring the gravity of precision in generative AI.
During Sam Altman’s visit to India in June, the CEO of OpenAI engaged in discussions with experts from various backgrounds.
When Altman reached the Indraprastha Institute of Information Technology Delhi, Professor Bagler took the opportunity to pose a vital question.
By expressing his concerns, Bagler shed light on an alarming issue: although minor hallucinations in ChatGPT may be tolerable, when a recipe produced by the AI system is similarly affected, it becomes a critical problem.
The professor’s insightful remark highlighted the need for continued refinement and improvement in generative AI systems, acknowledging the potential consequences of even slight inaccuracies in certain domains.
During a discussion on the topic, Bagler eventually posed the question, “What’s your take on it?” Altman responded with a sense of optimism, although he did not make a definitive commitment.
He expressed his belief that the issue of hallucination in language models would significantly improve in the future. Altman estimated that it would take approximately a year and a half to two years to reach this point. At that stage, discussions about hallucinations would no longer be necessary.
Altman emphasized the importance of finding a balance between creativity and perfect accuracy in these models. He stated that the model should be able to discern when one is preferred over the other.
However, for experts like Bender, who have extensively studied the technology, these improvements may not be sufficient. Bender defines a language model as a system that models the likelihood of different word forms based on written data it has been trained on.
Spell checkers play a vital role in detecting typographical errors and wrong word choices in written text. Moreover, they contribute to the functionality of automatic translation and transcription services, enabling the generation of output that closely resembles the natural language of the target audience.
The widespread use of this technology is evident in various applications, such as the popular “autocomplete” feature incorporated in text messaging and email platforms.
Although modern chatbots like ChatGPT, Claude 2, and Google’s Bard attempt to elevate text generation capabilities to new heights, they still rely on repeatedly selecting the most fitting word within a given context.
Language models, when employed for text generation, are fundamentally designed to fabricate content. They exhibit proficiency in emulating different writing styles, encompassing legal documents, television scripts, or even poetic sonnets.
However, it is crucial to note that these models solely rely on chance when producing interpretable text. Even with optimization, they are prone to failure, particularly in obscure scenarios that may evade easy detection by human readers.
Despite these limitations, companies in the marketing industry, such as Jasper AI, find these errors to be inconsequential in their pursuit of refined pitches and narratives, as affirmed by Shane Orlick, the company’s president.
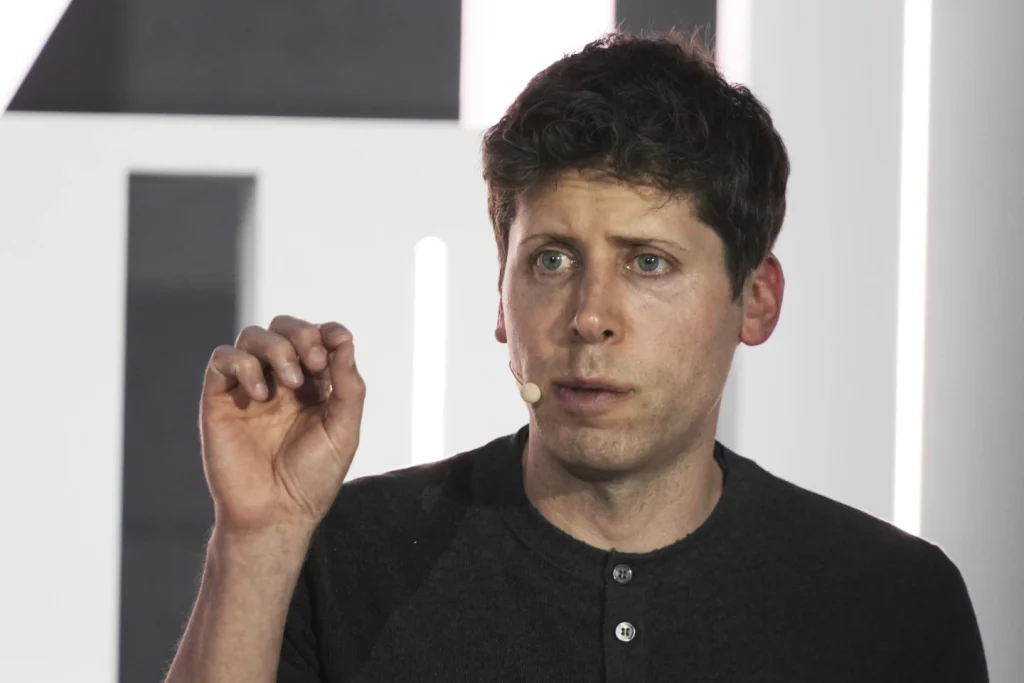
According to Orlick, the founder of a Texas-based startup, hallucinations in AI language models are not necessarily a drawback, but rather an additional advantage.
Customers often praise the innovative ideas and unique perspectives that these models, particularly Jasper, bring to the table. To meet the specific requirements of their clientele, the startup collaborates with prominent partners, including OpenAI, Anthropic, Google, and Meta, tailoring its AI language models accordingly.
For instance, customers concerned with accuracy might be directed towards Anthropic’s model, while those prioritizing data security may be recommended a different model.
While Orlick acknowledges that fixing hallucinations won’t be an easy task, he remains hopeful that influential companies like Google will dedicate significant efforts and resources to finding solutions.
Recognizing Google’s commitment to ensuring factual content on its search engine, Orlick believes they must address this issue. While perfection may be unattainable, Orlick anticipates that AI language models will continue to improve over time.
While some techno-optimists, such as Bill Gates, believe in the potential for AI models to be trained to differentiate between fact and fiction, others, like Altman, remain skeptical.
Altman even humorously expressed his lack of trust in the results generated by ChatGPT during an event at Bagler’s university.
Despite varying perspectives on the matter, Gates cited a 2022 research paper from OpenAI as evidence of the promising advancements being made in this field.